4 things you need to know about digital twins in the water industry
During the Singapore International Water Week, the digital transformation of the water industry was a hot topic. Industry leaders from all over the world shared case examples and thoughts about this disruptive digital revolution. I call it disruptive because digital twins will revolutionise plant operations.
In this blog article, I'm summarising the main takeaways of the 8h-workshop on 'Digital Transformation of the Water Sector and Role of Digital Twins', including the value of digital twins, the big shift in how we will use models and the confusion and debate around the definition.
1. What is the value of a digital twin? Discover the three major benefits
Let's start with the essential point: WHY? Because digital twins began to emerge just recently, there is a current lack of tangible case examples. Therefore I will first list three cases Ifrom the workshop and distil the generic benefits.
Jesper Kjelds (Aarhus Vand) stated that "Digitalisation is not a goal on its own. It is a means to reach business goals".
Before we dive into the benefits, I heard about two essential side effects of digitalisation:
- As digitalisation is a way to reach business goals, a digitalisation exercise is a powerful way to reassess, review and communicate business goals (which are often not clear to everyone).
- Digitalisation is a change management exercise. We need change anyway with the current challenges we're facing (e.g. climate change). So it's good training in resilience for many people.
Three practical case examples
While participants showed more examples, the following three were very tangible:
- Reducing non-revenue water in drinking water distribution in the Valencia area (Spain) through 'leak prioritisation' (i.e. which leaks to fix first) based on machine learning (Global Omnium, Spain)
- Enhancing the stability of membrane bioreactor (MBR) operation through advanced data analytics and machine learning (PUB, Singapore)
- Highly accurate 1-day prediction of influent flow using artificial intelligence, leading to smoother downstream plant operation (Clean Water Services, US)
The benefits summarised
Multiple speakers came with figures summarising the main benefits of digital twins or digitalisation in general (Figure 1). There are' human benefits next to direct operational benefits (i.e. plant performance and efficiency). The first is critical as digitalisation is a change management exercise, and people need to see the benefits to go with the change.
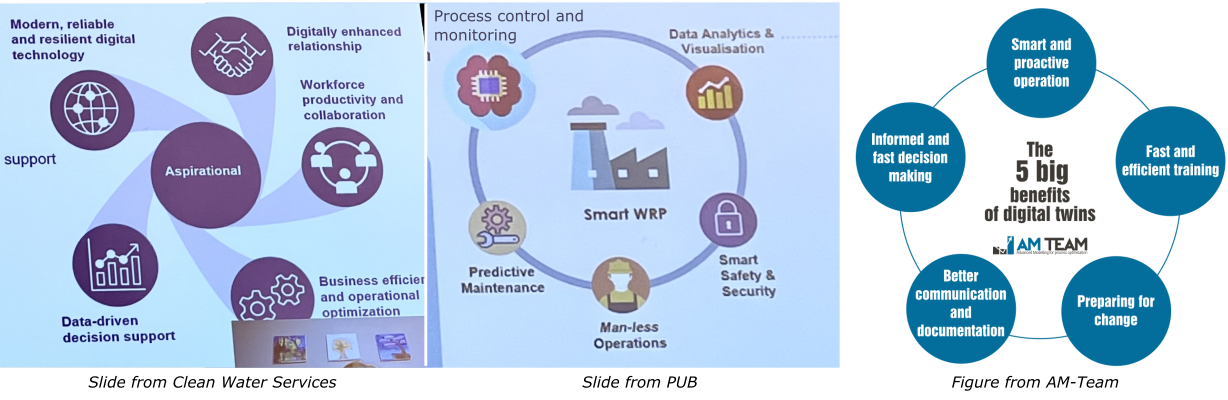
Figure 1: the 'benefits' of digital twins, summarised by three different organisations - Clean Water Services and PUB showed the first two figures in the workshop
One attendee asked: "How to convince management if the main benefits are not directly monetary of nature?". The discussion revealed the importance of non-monetary benefits. And the non-monetary benefits are mainly people benefits. But, of course, one benefit can be in several categories. For example, 'the storage of experience from an ageing workforce' in a digital twin leads to monetary and people benefits.
While we can identify 5 to 8 (e.g. Rasheed, 2020) distinct benefits, I could distil the following three big categories from the workshop:

Figure 2: monetary (and environmental) benefits belong to the first category, but many non-monetary benefits relate to humans
2. From 'disposable models' to 'models for permanent use'
The digital twin revolution means the increased use of models in the operational stage. But it does not mean that models in the water industry are new. For example, simulation models have been used to design wastewater treatment plants for decades (I think the first simulations were ongoing when I was not yet born).
HOWEVER, here's the significant change:
Models used to be applied in the planning and design stage only, after which most of them got archived once the plant started operating.
The digital twin revolution stimulates the 'reuse of models' throughout the technology lifecycle.
We typically define the process technology lifecycle as planning, design, construction and operations/maintenance. However, at AM-Team, we even look at the earlier step of novel tech development in the R&D stage.
We have examples where models started in the early R&D stage (e.g., accelerating scale-up of a new bioprocess technology) while moving up the value chain (as the tech vendor is now commercialising the novel technology). Ultimately, the same model will end up as a digital twin.

Figure 3: the technology lifecycle and the use of models per stage, as typically presented by AM-Team. People have different ways of presenting them, but the core elements are similar: from 'preparation' to 'operation'.
Here's the beauty: while models of the design stage used to be archived, you can now reuse the same models for operation. So even though model upgrades/ enhancements might be needed, as real-time use is challenging, we can talk about an increase in the 'use of modelling resources'.
3. Starting with a twin baby is the best way to start :-)
Multiple people stressed the importance of starting small. Like real twins begin as babies, it's a smart idea to move forward in baby steps. For example, starting with a digital twin of a small part of your process train, based on the most acute needs. Then, use those 'victories' to demonstrate the value and take it from there. While it can be the vision, the all-encompassing 'beast model' might be too intimidating.
Baby stepping the process is a very good strategy for many of our customers.
Bruce Johnson (Jacobs)
We agree. At the moment, we're building a digital twin for an advanced treatment plant for micropollutant removal. While the whole plant would benefit from a digital twin, starting with a 'sub-digital twin' of the ozonation process only will lead to tangible value and a showcase.
Of course, going step by step also brings 'integration challenges'. One of the attention points for the coming years will be assuring the 'compatibility' between different digital twins if you want to combine them in the future. It means compatibility of models, but also concerning data. As Udugama et al. (2020) states, data formats will matter in the future.
A great objective for 'data scientists' is assuring this compatibility. So yes, more 'standards' will be needed. But let's start with some tangible great cases first.
Here are the main building blocks of a digital twin, nicely visualised by SWAN (Figure 4).
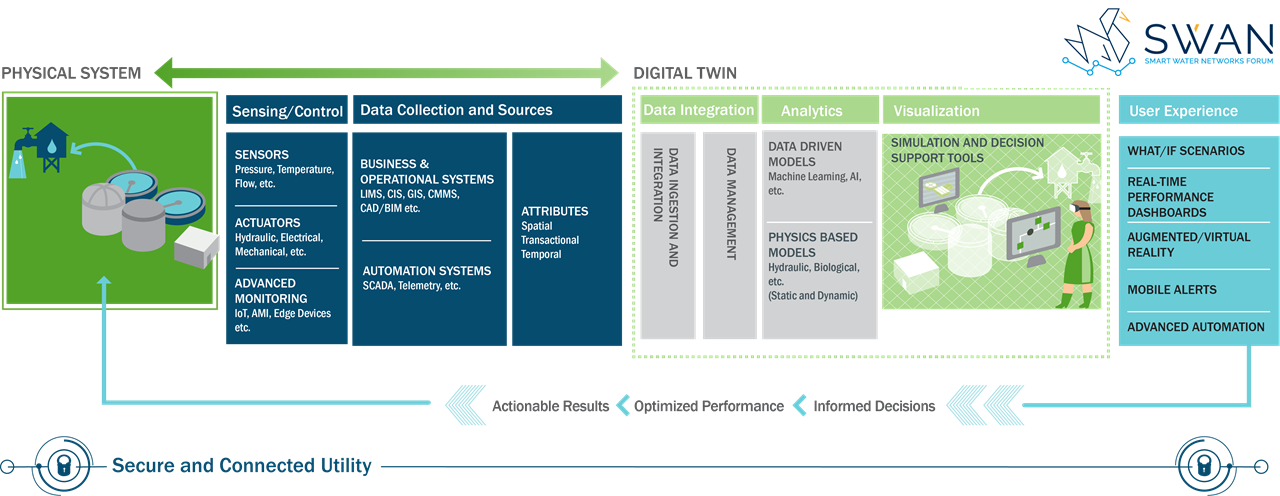
Figure 4: the digital twin architecture by SWAN showing the 'real' and 'virtual' worlds, and the user experience
5. What is a Digital Twin?
Let's end with the definition of 'digital twin'. While everyone agrees about its digital nature, not everyone agrees that a digital twin needs a 'real-time' data flow. To some people (e.g. according to the definition by SWAN),a model used in the design stage prior to real plant construction, can also be called a digital twin (Figure 5).
However, as Udugama et al. (2022) stated, we have to be careful not to 'sell old wine in a new bottle'. As I stated earlier, wastewater simulation models have been in use since the late 80s.
Reviewers have pointed out that aspects of digitalisation can be like “old wine in a new bottle”, and so identifying the misuse and incorrect use of terminology can be helpful. The term digital twin in the processing industries is often, quite naturally because it is a developing space, incorrectly used interchangeably with digital simulation models.
Udugama et al. (2022)

Figure 5: the technology lifecycle and the 'two big groups' of people with different definitions - however, what's most important is that both groups talk about 'reusing models throughout the value chain'
My personal opinion
I will be honest, at first, I was also confused, searching for my definition. However, my thoughts seem to have crystallized after consulting some key literature in the last couple of weeks. I agree that the 'real-time' component is the novelty and power of a digital twin, not to be confused with 'simulation models' in an earlier stage. To me, you can't talk about a twin if only the virtual baby brother or sister is born. The reason why I think 'digital twin' must be something new is because we have key enablers that were not available before: massive computing power, the magic of (mobile) internet and great data analytics.
A digital twin is a model application we did not have before. And the challenges and the value proposition of the 'real-time dimension' are completely different. It's the difference between a 'regular plant' and an 'intelligent plant'.
Let's have a look at the conflicting opinions:
Definition by SWAN
A dynamic digital representation of real-world entity(s) and their behaviours using models with static and dynamic data that enable insights and interactions to drive actionable and improved outcomes
Definition by Udugama et al. (2022)
A digital representation of a physical system that looks like, behaves like and connects to that physical system
Definition by Rasheed et al. (2020)
A virtual representation of a physical asset enabled through data and simulators for real-time prediction, optimization, monitoring, controlling and improved decision making
At AM-Team, we typically use 'digital twin' when a model is applied in the operational stage (see Figure 3).
Conclusion
The future is bright. We have big challenges ahead, but we have beautiful digital tools, models and computers. Regardless of the definition, everyone seems to agree that we can use this digital power to make a better world. So seeing it from that perspective, the definition matters less.
Interested in more? Read these blog posts
- Make your pilots virtual: three ways how Computational Fluid Dynamics (CFD) simulation improves your process design and scale-up
- Unravelling the spatial secrets of N2O by combining advanced modelling tools
- The wastewater and drinking water worlds: two continents drifting towards each other
Thought leaders from the workshop
Albert Cho (Xylem), Amir Cahn (SWAN), Andrew Shaw (Black & Veatch), Min Zhong (NEOM), Ting Lu (Clean Water Services), Tao Guihe (PUB), Ridzuan Ismail (PUB), Miguel Ángel Ayllón Mesa (Global Omnium), Melvin Koh (PUB), Maree Lang (Greater Western Water), Jesper Kjelds (Aarhus Vand), Jennifer Rebeiro (Greater Western Water), Gigi Karmous-Edwards (SWAN), Eric Skowron (ProjectMax Ltd), Chengzi Chew (DHI), Andy Smith (Anglian Water Services), Agnethe Pedersen (VCS Denmark), Bruce Johnson (Jacobs), Sandeep Sathyamoorthy (Black & Veatch)
Acknowledgements
SWAN: thank you for setting up this wonderful workshop. I know, I have been critical about the definition, but at least you are trying to come up with a unified definition for the water industry, which is a very important initiative. It's easy to criticize work if you're standing at the sideline.
References
- Udugama, I., Bayer, C., Baroutain, S., Gernaey, K., Yu, W., Young, B., 2022. Digitalisation in chemical engineering: Industrial needs, academic best practice, and curriculum limitations. Education for Chemical Engineers 39, 94-107
- Rasheed, A., San, O., Kvamsdal, T., 2020. Digital twin: Values, Challenges and Enablers From a Modelling Perspective. IEEE Access 8, 21980-22012
Subscribe to our newsletter
