Digital water: from hype to value and action
During the last few years we noticed a significant acceleration in the debate around ‘digital water’. The talking is now rapidly transforming into action, certainly post covid and further fueled by the latest developments in artificial intelligence. Even though many water utilities and other water industry stakeholders are still learning about what digitalization is and means, concrete actions are being taken and vast budgets are being allocated.
In the coming decade, we expect a maturation of the digital water market, with game-changing applications entering the stage. Why? Because we need to look at solving some key challenges, as shown in Figure 1. Never before, these challenges coincided so much and became acutely felt.
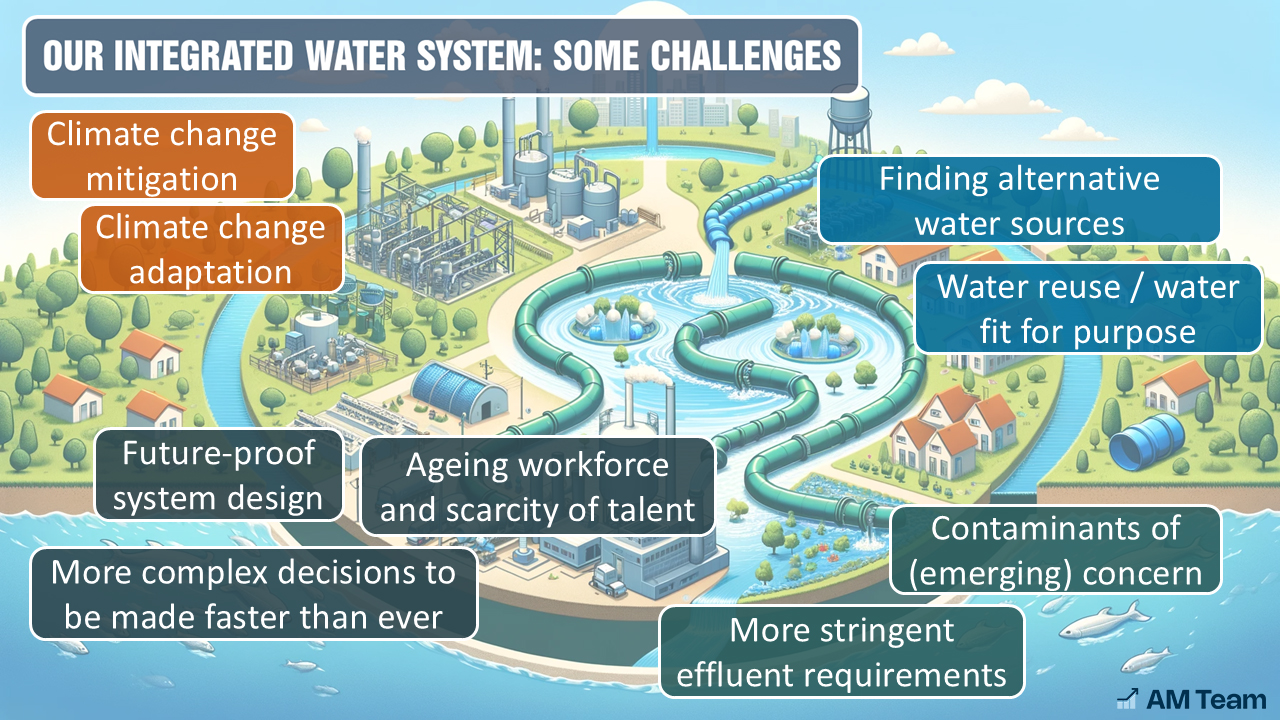
While digital water can be broken down into multiple components, an important group of components are ‘models’. These models describe and predict the behavior of our (many) assets. This article aims at further educating our market on basic principles of those models, as education is foundational to adoption of new technologies. The aim is to provide a helpful framework, rather than confusing with details.
The business case of digital water
Digitalisation is both hype and real. For sure, the talking currently outweighs the action and fancy terminologies are being used. What we specifically are interested in is looking beyond the hype.
The key question is what makes the business case of digital water and what is causing the business case to become a so-called no-brainer. Yes indeed, digitalization will not be a nice to have. In the coming decade, it will affect our core business.
We have been thinking about what would drive the business case of digital water. Figure 2 may provide a helpful framework. A business case becomes real if two items coincide:
- Challenges or pains that are being felt acutely or will be felt acutely
- Solutions that can solve those pains or will be able to solve those pains
Digital water is where challenges meet solutions. This pairing will dramatically grow in the near future and hence, the business case of digital water will become too strong to neglect.

This figures avoids that we look too narrow and as such miss opportunities. Throughout the years, we noticed that too often, digitalization is narrowed down to only parts of this framework. For example, ‘digital water is artificial intelligence’, or ‘digitalisation is leak detection’ or ‘digitalisation is all about smart plant operation’. Tools, challenges and business case are being mixed up and a complete overview of the potential is missing.
While the aforementioned items are all relevant, they only reflect parts of the whole.
The 5 business case categories
We identified 5 key families of business cases, shedding light on the vast potential of digital water.
- Smart and proactive operation: this is all about optimization of already existing assets, and most often ‘3E’ optimization1 (Effluent, Emissions, Efficiency). It is about meeting effluent goals, saving costs and reducing carbon footprint.
- Informed and fast decision making: this is all about using new digital tools and models to guide short-term decision making. For example, using a model to compare 2 operational strategies, while in the past this decision would be taken based on experience or simple rules of thumb.
- Preparing for change: this is all about using new digital tools and models to guide mid- and long-term decision making. We often call this the ‘crystal ball’. For example, using a model to anticipate future changes in influent load, or assessing climate change scenarios to make an adaptation plan. This functionality includes ‘non-existing assets’ (i.e. planning and design stages).
- Better documentation and communication: this is all about internal collaboration. Many utilities are big organizations. A key trend in the coming decade will be the use of tools across the organizations, with interfaces tailored to the users and their respective goals.
- Fast and efficient training: remember Figure 1 – the workforce challenge is one of the biggest challenges of our water industry. New models and tools will store experience on one hand, and will accelerate training on the other hand.
It now becomes clear that the value of digital water knows many dimensions. Yes indeed, the business case is becoming very attractive, as it contains both ‘hard gains’ (e.g. ‘meeting effluent goals with minimal cost’) and ‘soft gains’(e.g. ‘empowering people to make better and faster decisions’), and is not only limited to operations but also includes (early) planning and design.
The 3 big families of models
Now, let’s zoom in a bit. First look back at Figure 2: key technological building blocks are models (such as AI and mechanistic models). Only few people in our industry today know the big families of models (Figure 3), causing a lot of confusion.
Clearing those misunderstandings might lead to better decision making, will avoid some application mistakes and ultimately will lead to faster and more successful adoption of digitalization.
- Mechanistic models: we like to call those models ‘knowledge-driven models’, as they are based on known laws of physics, chemistry and biology. These models hence have ‘a priori knowledge’ (i.e. they know things on beforehand, even before they have seen any data or plant). As the equations and their parameters have a physical meaning, they can be called ‘white box’.
- Data-driven models: these models are trained based on available data. They can be fedwith huge amounts of data, and many different variables and will predictpatterns and trends based on that information. As we do not know how they dothat, they can be called ‘black box’.

Successful and promising applications of data-driven and mechanistic models
What is often not well understood in our industry is that these tools are fundamentally very different, and each of those categories have their strengths and weaknesses. Figure 4 shows some of the key domains of those models as of today. For example, data-driven models are being used at large scale for leak detection (e.g. feeding pressure data to ML algorithms) or asset management (e.g. feeding pump vibration data to an AI model to predict the health). Knowledge-driven models are often used at the treatment side (e.g. a mechanistic model predicting the effluent nitrogen in a water resource recovery facility based on incoming ammonia dynamics).

To provide you with some more concrete examples on each of those, we have included Figures 5 and 6. Notice that data-driven does not only entail AI or ML. It also entails statistical models that have been established for many decades.

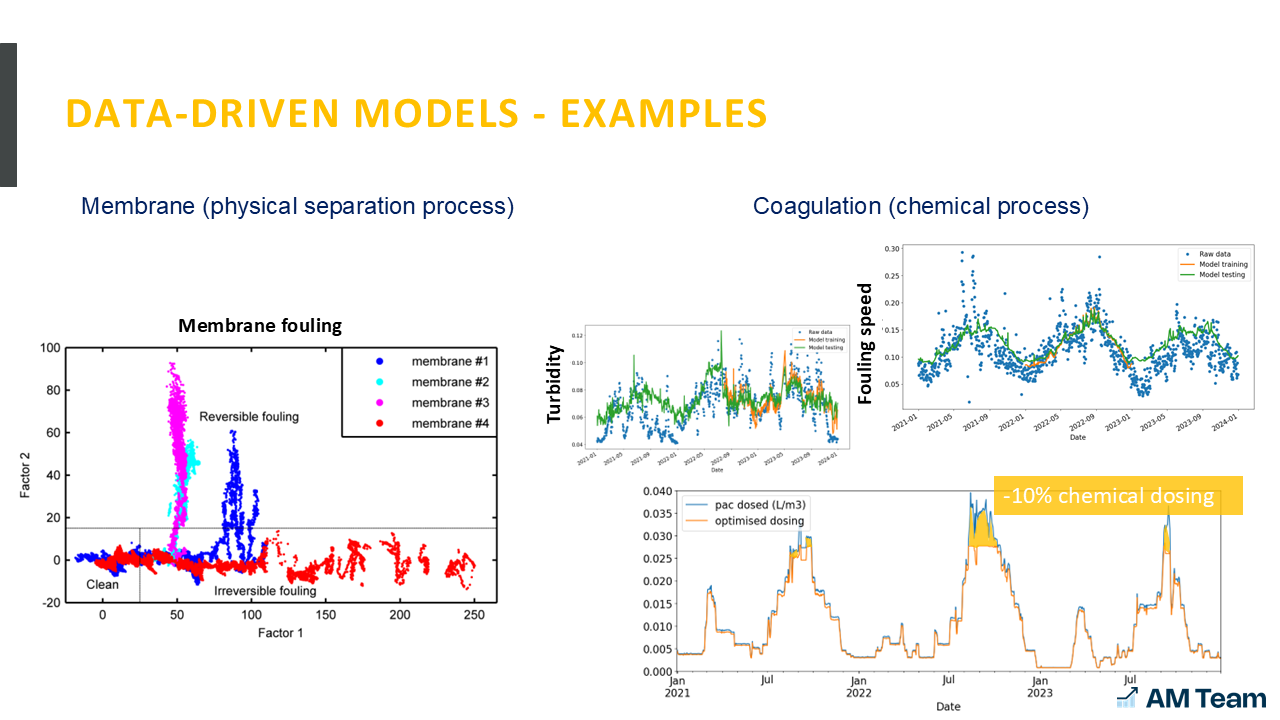
T. Maere et al. Membrane bioreactor fouling behavior assessment through principal component analysis and fuzzy clustering, Water Res. (2012)
The strengths and weaknesses of the different models
Just like with a team of people, each individual has strengths and weaknesses. Composing a dream team consists of letting people work on theirs strengths as much as possible. Let’s look at some of the key strengths and weaknesses of the two big families of models.
Figure 7 reveals that the model families are in fact no competitors but have great potential for a dream team, as their respective strengths and weaknesses are complimentary.

The future of digital water is a toolbox
A key feature of digitalisation in the coming decade will be a massive integration of those models into the core business of utilities. While the models have been used before (e.g. mechanistic models have been used to design plants for decades), the context of their applications will dramatically change (see Figure 2 – the different business case items).
Knowing that, it will be essential to recognize that no one tool can solve everything and that a smart combination of tools will be the key to success. Either both families can work alongside each other (e.g. a data-driven model predicting what’s coming into a plant based on weather forecasts etc. while a mechanistic model predicts the plant’s performance itself), or can be integrated, indicated by the hybrid category in Figure 3 (e.g. a data-driven model making sure a mechanistic model stays on track by automatically calibrating some of its parameters).

Only sufficient education can let us use the right tool for the right purpose. We all know you can hammer a screw, and it even might get in. Yet, we all know it’ll be not optimal and beautiful. Let us use a hammer for a nail, and a screw driver for a screw. And let us realise we need both screws and nails, and hence a toolbox to reach our goals.
Blog post written based on AM-Team’s CEO Dr. Wim Audenaert’s keynote lecture at the IWA Global Water Summit 2024 (Bilbao, Spain).
1 In2022, AM-Team proposed the 3E framework to summarise the key challenges and complexity utilities face. Each E is a juggling ball, and today’s water and wastewater treatment is a juggling act.
Suggested insights
Subscribe to our newsletter
